Sensefy – Content Discovery Through Entity Driven Search
Leveraging enterprise information is no easy task
Especially when unstructured information represents more than 80% of enterprise content. Meaningfully structuring content is critical for companies, Natural Language Processing and Semantic Enrichment is becoming increasingly important to improve the quality of tasks related to information retrieval.
With the Semantic Web moving towards full realisation thanks to the Linked Data initiative and with the interest of major search engines in structured data, the enterprise search world is finding it more attractive to make its information machine readable and exploit that information to improve search over its content.
Three trends are transforming the face of search:
1. Entity-oriented search. Searching not by keyword, but by entities that represent specific concepts in a certain domain.
2. Knowledge graphs. Leveraging relationships amongst entities: Linked Data datasets (Freebase, DbPedia….) or custom companies’ knowledge bases.
3. Search assistance. Autocomplete and spellchecking are now common features, but making use of semantic data makes it possible to offer smarter features, guiding the users to what they want, in a natural way.
Sensefy
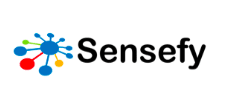
In this scenario comes Sensefy, the new Semantic Search Engine developed by Zaizi; based on cutting edge Open Source technology it will bring a complete new search experience to users.
Architecture
The key point of Sensefy is flexibility:
Starting from the input, the system allows to index data from a wide set of heterogeneous data sources, making possible a federated centralised search on this information.
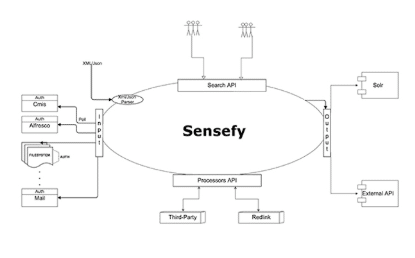
- Alfresco
- CMIS
- DropBox
- File System
- Google Drive
- HDFS
- Windows Shares
- JDBC
- Jira
- RSS
- Web
- Wiki
- LiveLink (OpenText)
- Documentum (EMC)
- SharePoint (MSFT)
- Meridio (Autonomy)
After the information extraction phase, the retrieved documents will be processed.
Sensefy offers an highly configurable environment where the administrator can manage the semantic enrichment from high level to the deep details.
Sometimes, the proper resources to provide advanced search on a document corpus are not easy to obtain. In order to generate these, our approach includes a number of unstructured data processing mechanisms the goal of which is to automatically extract semantic information:
- Extract content from heterogeneous data sources
- Extract domain information and enrich the content through different NLP processes like Named Entity Recognition, Coreference Resolution, Entity Linking and Disambiguation, and Topic Annotation
- Create specialised indexes to store the semantic information extracted
To provide this, Sensefy leverages the power of Redlink, a “Software as a Service” (SaaS) running on a distributed cloud infrastructure.
Redlink Content Analysis offers fact extraction, topic classification, and fact linking from textual and media documents in different languages. Users are able to send text (and in the future media content) and get back facts and entities that have been identified automatically. In addition, the service provides references to existing datasets (public datasets such as Dbpedia and Freebase, as well as custom user-created vocabularies such as a product database) about the entities identified in the content.
And this is a key point : Custom Datasets.
Everyone can easily configure their knowledge base to customize the semantic enrichment of their documents to obtain very precise and domain specific enhancements.
Currently there are a number of well developed uses of semantic extracted information such as faceting and concept indexing, however further methods of exploiting semantic extracted information are presenting themselves in the industry:
Smart Autocomplete
The target of this feature is to automatically complete users’ phrase with entity names and properties, helping them to find the desired documents through exploration of the domain Knowledge Graph. As the user keys in the phrase, the system will propose a set of named entities and/or a set of entity types. As the user accepts a suggestion, the system will dynamically adapt following suggestions to the chosen context.
Important is the support for multi-language entity type autocompletion , so while users are typing in their own language, the system will recognize the concept independently of the way is expressed ( alias are supported as well).
Once a type is selected the user can proceed in building a query driven by the context, specifying the attributes for the concept selected in a natural way.
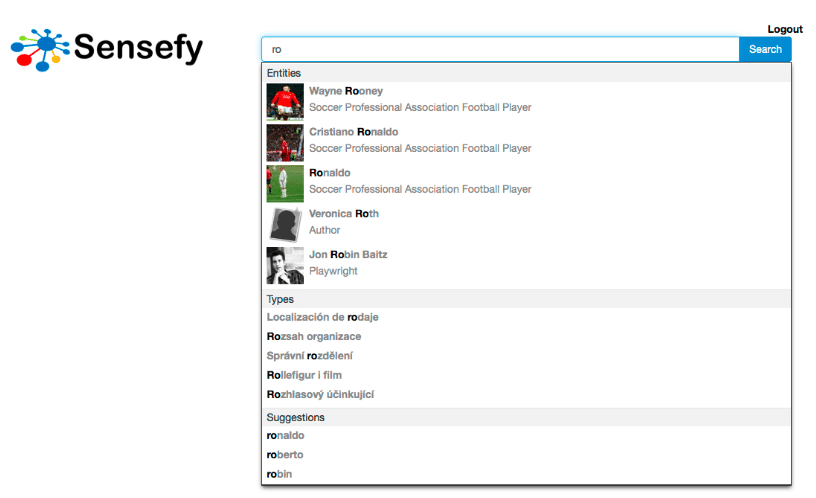
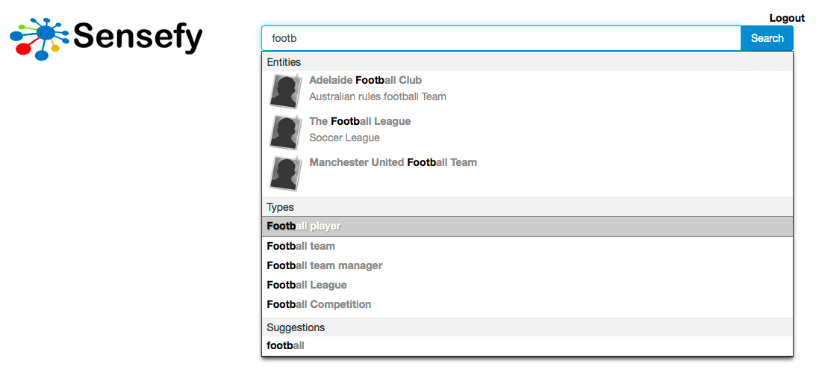
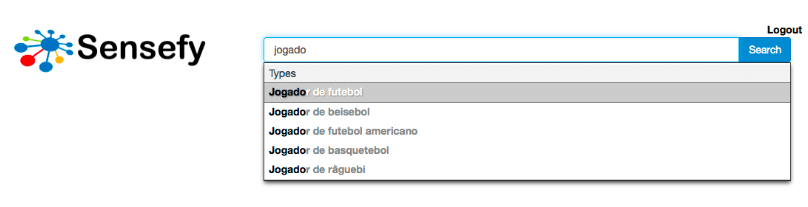
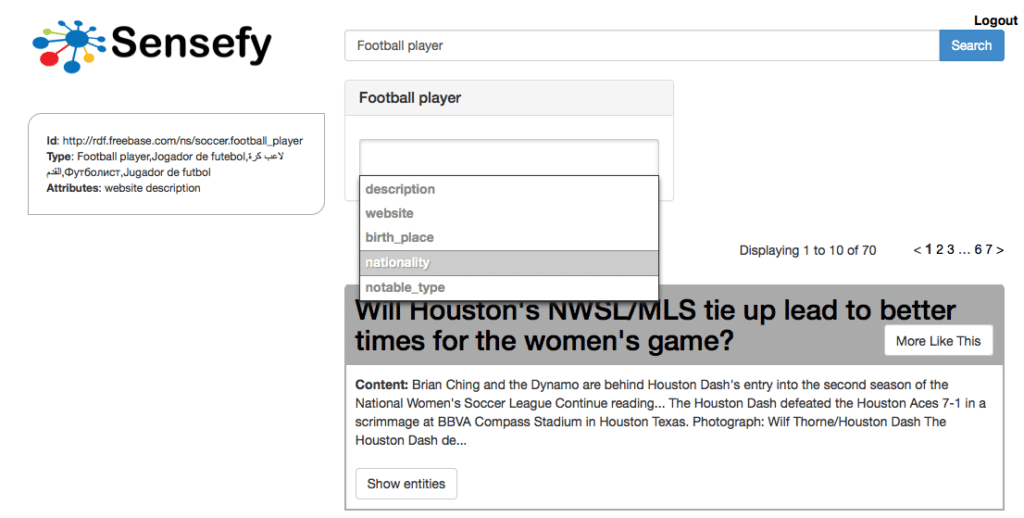
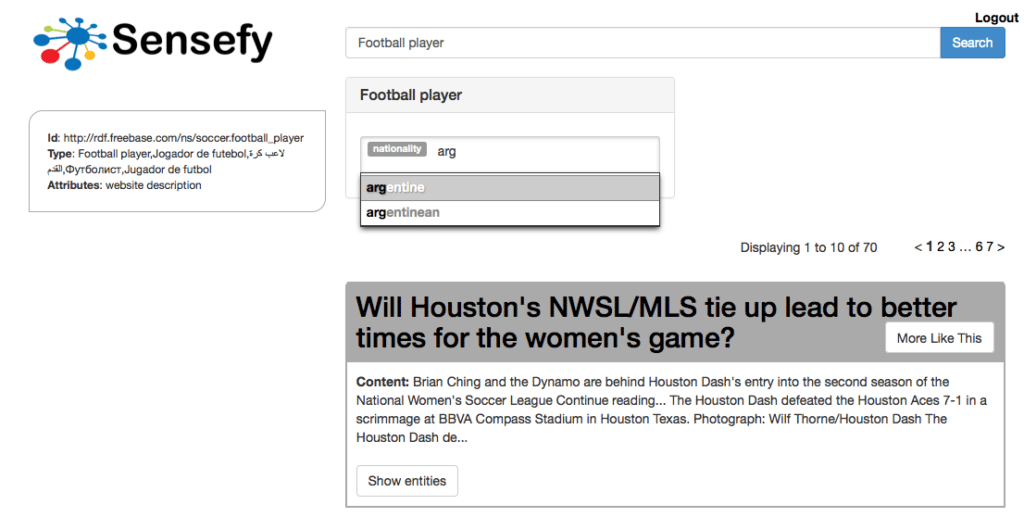
The accuracy delivered by entity driven search brings increased satisfaction among users. They will see documents that are about a specific semantic concept, with concrete properties, and not about a keyword that can be ambiguously interpreted.
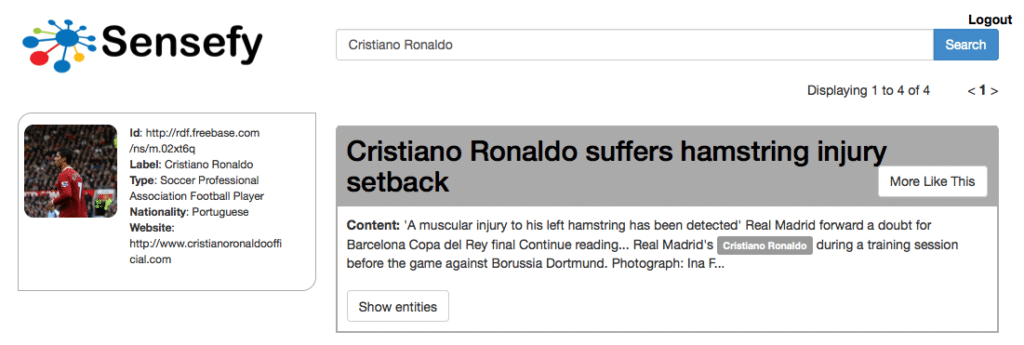
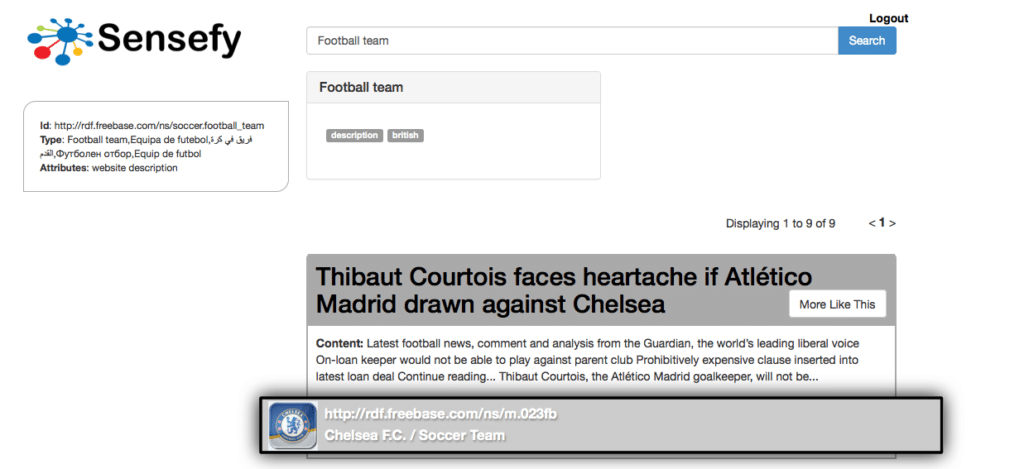
Semantic More Like This
A feature to find documents similar to a seed one, based on the underlying knowledge in the documents, instead of tokens.
Implementing a semantic distance function, we can provide a grade of similarity between documents based on the concepts and entities inside those documents. These techniques take into account the actual relations between entities, not only within a document, but also in the whole Knowledge Base. So, even if two documents are not sharing any entity, they could share a common topic (they would have the same semantic background) because all the entities are close to each other in the Knowledge Base Graph (locality).
The strength of this approach is the chance to retrieve similar documents by sense , so even if two documents are not sharing any term, maybe they share different entities of the same or related entity type. In this way we can correct a lot of false negative matches that the classic term based approach can bring.
The feature is shown with a slider that allows the user to scroll from a strict selection of similar documents to a wider one.
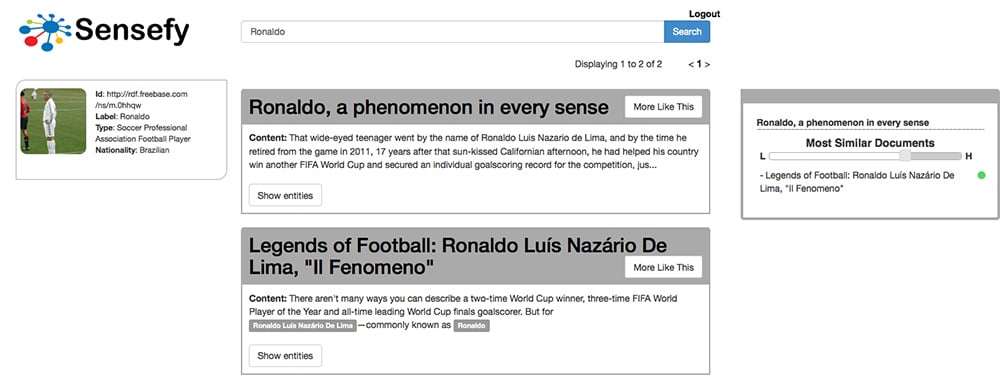
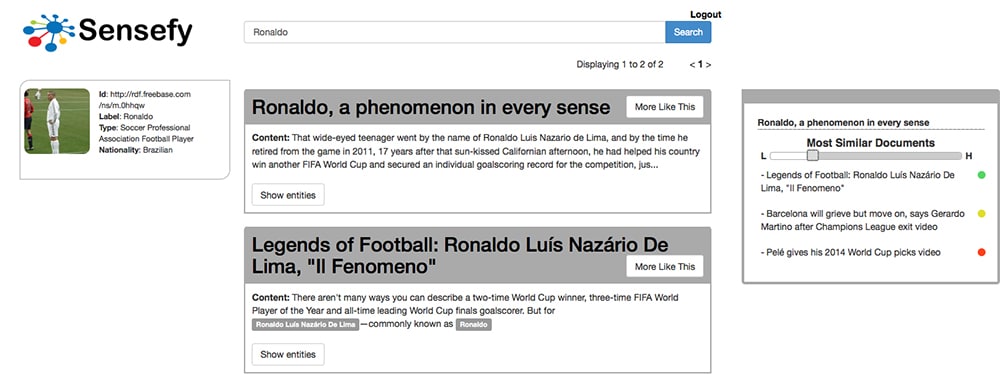
Latest content
-
Paris AI summit: Navigating the AI landscape and how SMEs can help UK government
Published on: 19 February, 2025 -
Border Force wins Global Customs Innovation Award for ScanApp — a solution Zaizi helped build
Published on: 14 February, 2025 -
How Zaizi’s user-centred approach won the trust of border officers
Published on: 17 December, 2024 -
Does the state need to be more like a start up?
Published on: 12 December, 2024