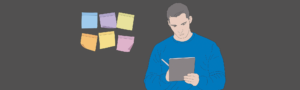
Discovery to alpha: Mastering ambiguity in digital public service delivery
You’ve completed discovery and decided you’re ready to move to alpha in your digital public service delivery. But as GDS puts it, the reality is you’ve only established whether “there is a viable service you could build.”
The key word here is ‘could’. I know, it’s tempting to feel like you should have all the answers or find them all in alpha. But alpha means you’re still in an exploratory phase. You still need to test the riskiest assumptions unearthed during discovery.
You should also still be exploring opportunities to improve things compared to how they’ve been done in the past. For example, is there benefit from sharing data with other teams or departments?
It’s crucial not to get frustrated by the lack of clarity at this point. I do get the angst. Time is short and you may well be under pressure to move quickly and prototype the entire user journey.
But living with continuing ambiguity is an essential part of the alpha phase. Essentially, you’re working through the bit between understanding what your problem is, and knowing how you want to solve it.
So how to manage this? In this post, we share how we approach this uncertain but fascinating phase of digital public service development at Zaizi.
Our objective – as always – is to help you mitigate the risk of building the wrong solution that doesn’t solve whole problems. Or just as damaging, incurring significant extra development costs down the line.
Stick to tried and tested product management principles
Our approach works in line with the three well-established pillars of product management:
Desirability: We start by looking at what the greatest risks are to user adoption. These are likely to be specific parts of the journey – such as what the best sequence of steps will be to request payment within the journey that avoids drop-offs.
Feasibility: We look closely at whether it’s possible to build out the digital public service we’re developing in a sustainable way. For example, if we think we’ve identified the journey that users want – and we also believe we might need access to a data set that hasn’t previously been integrated – we’ll investigate whether that will be technically feasible.
Viability: Crucially, we also consider how we can achieve the aims of our clients within the constraints of legislation, policy and budget. So taking that data integration example again, we might look at whether the relevant data-sharing agreements are in place. Or if not, whether a change in legislation or policy will be required to get those agreements. Only then will we know if we need further work to find a viable solution.
Principles in practice – how we identify the risky areas on real projects
Our process is made up of three elements:
- We draw on user-centred design techniques to map out our current understanding of the end-to-end journey at the right level of detail.
- Our multidisciplinary team (made up of designers, researchers and solution architects) run through the risks from different perspectives across the three dimensions of product management.
- We then prioritise the riskiest areas and agree the approaches to further our understanding.
Here are some examples of how we’ve applied elements of this approach to real digital public service design projects.
READ: How to design the best service design team for digital public services
- Assessing experience
Recently, we worked on an alpha for a concessionary travel scheme. Our discovery identified challenges in how users applied for the scheme and managed their accounts. We could see there was still a significant conundrum to solve: users wanted digital solutions that would allow them to self-manage the process, but they lacked confidence in using them.
This led us to identify two key parts of the journey that were critical to adoption and carried the most risk:
- Users consenting to data sharing around access to blue badge information – we knew this would significantly improve the experience, but realised the real challenge would be explaining the benefits in a way that encouraged users to give us access.
- Users providing evidence of identity – a higher proportion of users had specific accessibility needs, which meant they had difficulty providing identity details such as uploading passports or taking images of themselves. Our discovery research identified a range of solutions that government departments had used to address this issue before. But we weren’t clear which would be the best for our users.
To remove these areas of ambiguity, we prototyped and tested approaches for both of these risky areas. Only then did we have the confidence to take forward the best solutions into beta.
- Assessing feasibility
Another recent discovery showed that bringing together numerous sources and formats of documents (e.g images and PDFs) was critical to the success of the service we wanted to create. Some of these sources were tried and tested, so we weren’t too worried about them. However, there were two specific sources that hadn’t been aggregated before. This meant we struggled to quantify the quality of the data and our ability to match customer records across these data sets.
In alpha, we carried out technical investigations to find out whether we could automatically score and prioritise the submitted document – so that only low-scoring items required manual inspection.
To test the feasibility of this, we created a data pipeline in Amazon Web Services that used AI services to extract data from images and PDFs. We then ran a custom scoring algorithm against it.
This gave us the appropriate level of confidence that we could technically enable this critical part of the journey. It also meant we had an acceptable level of certainty that validated both (a) our technical approach, and (b) the likely operating model required to support the document verification we needed.
- Assessing viability
We also recently completed a discovery into the support that leaseholders get when they are trying to resolve disputes. The exercise identified a number of strengths with current approaches. However, we identified some limitations that we were unsure we could resolve within the budget for the service.
In alpha, we decided to model the various options to make the greatest use of the budget to maximise positive outcomes. This involved looking at current and predicted future demand against a range of potential digital and non-digital solutions to find the perfect balance. Alongside this, we carried out user testing to allow us to make informed assumptions about potential uptake.
As a result, we could prioritise and move onto the delivery phase to implement the solutions with the highest potential for positive impact and deemed feasible within the available resources and timeline.
Conclusion
The most important thing to keep in mind during alpha is that you’re trying to de-risk building a service.
You’ve moved towards the point where the cost and stakes are higher – because soon you will start to commit to a larger investment in terms of people’s time.
The examples we’ve highlighted here also show the value of a multidisciplinary team that can view risk in alpha from different angles and help build confidence that you’re solving the right problems. By focusing on the riskiest assumptions, you learn without getting too attached to a specific solution.
And all of this helps you avoid a costly, unanticipated pivot later in the process.
If you’d like to find out more about our work, please get in touch.
-
From ScanApp to Lego demos — What you need to know about Security & Policing 2025
-
Truth, trust and transformation — 4 key takeaways from Zaizi’s AI in government event
-
Zaizi talent shines at Computing DevOps Excellence Awards 2025
-
Advancing DevOps practices with AI —lessons from AWS re:Invent
-
Paris AI summit: Navigating the AI landscape and how SMEs can help UK government
-
Border Force wins Global Customs Innovation Award for ScanApp — a solution Zaizi helped build